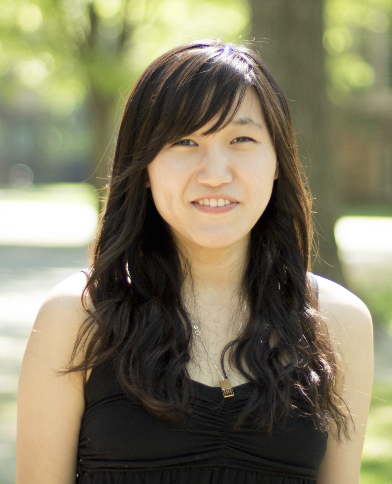
About
Lisa Lee is a Research Scientist at Google DeepMind. She completed her PhD in Machine Learning at Carnegie Mellon University, advised by Ruslan Salakhutdinov and Eric Xing. She graduated summa cum laude with an A.B. in Mathematics from Princeton University, where she was advised by Sanjeev Arora.
Research Interests
I am interested in building AI agents that can learn and adapt like humans and animals do, with the following desiderata: It perceives the physical world and acts according to its understanding of the world. It competes, cooperates, and communicates with other “living” entities. It is born with inductive biases accrued from billions of years of evolution. It keeps a memory of the past, and anticipates the future using learned biases. It performs abstract reasoning to plan ahead, learn higher-order skills, and learn concepts beyond the physical world. It continually adapts its skills and knowledge representation for better generalization. It is intrinsically motivated by curiosity, empowerment, and the need to be in control over itself and the environment.
I am most excited about bringing computer bits to “life”. When I was little, I felt an attachment to digital pets which are just binary bits in the computer; a snowman that I tried to save in the fridge one spring; and other non-living, not-necessarily-physical things. My childhood dream was to make Pokemon creatures real by building intelligent Pokemon robots. I still feel driven by the same childhood yearning.
Keywords: Deep reinforcement learning, intrinsic motivation, learning to explore, continual learning, hierarchical planning, multi-agent learning
Teaching
I genuinely enjoy teaching and advising students. My teaching style is inspired by my past instructors Claudiu Raicu and Robert Dondero from Princeton University, who helped reinforce my self-confidence and resilience in tackling progressively more challenging problems.
I was twice awarded TA of the Year for Deep Reinforcement Learning (2018) & Probabilistic Graphical Models (2019).
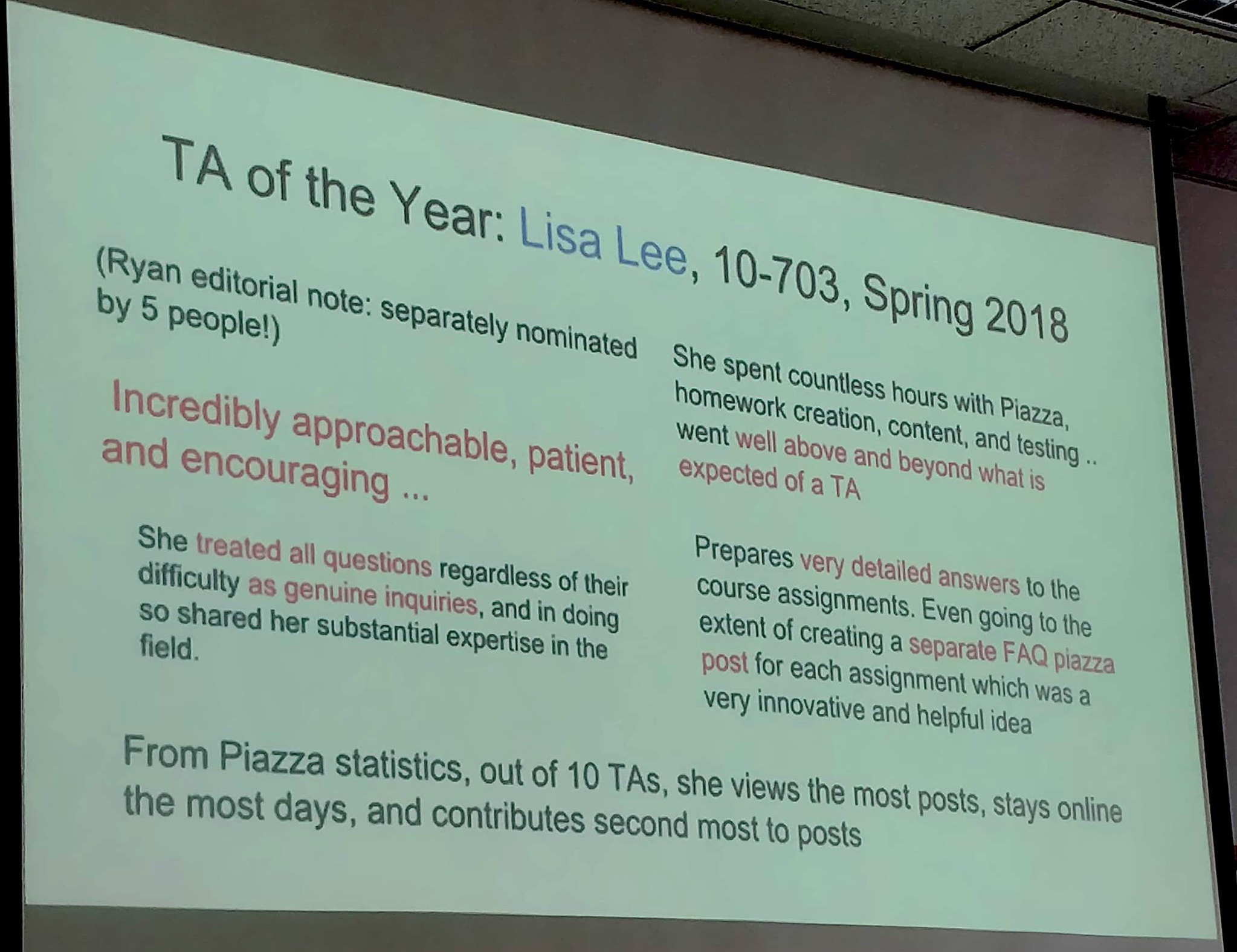
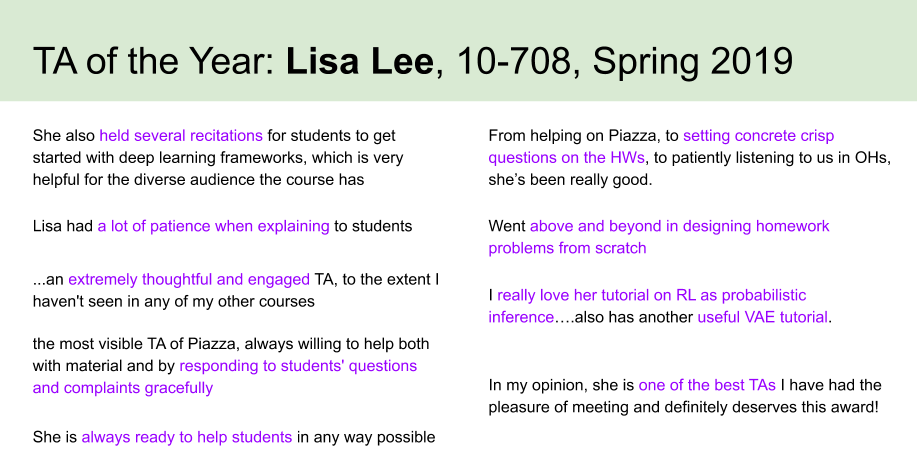
-
I was a TA for Honors Real Analysis (MAT 215), Honors Linear Algebra (MAT 217), Intro to CS (COS 126), Algorithms and Data Structures (COS 226), and Programming Systems (COS 217) at Princeton University.
-
I was also a TA mentor for Princeton Scholars Institute, a mentorship program for incoming first-gen and low-income students.
-
I also used to teach private cello lessons (2007-2011).
Conference & Workshop Committees
- I was a Workflow Chair for ICML 2019.
- I co-organized NeurIPS 2021 workshop on Ecological Theory of Reinforcement Learning (EcoRL): How does task design influence agent learning?.
- I co-organized NeurIPS 2019 workshop on Learning with Rich Experience (LIRE): Integration of Learning Paradigms (LIRE).
- I co-organized ICML 2018 workshop on Theoretical Foundations and Applications of Deep Generative Models (TADGM).
- I served as a reviewer for ICML (2021, 2020), ICLR (2021, 2020), NeurIPS (2020, 2019), CVPR (2018), UAI (2019), and NeurIPS Deep RL Workshop (2019, 2020).